The content on this page is intended to healthcare professionals and equivalents.
- * This article is based on interview with Mr. Junya Morita, who is a devleloper of Positining Analysis.
- * Positinig Analysis is an option for Fujifilm Digital Mammography, AMULET SOPHINITY
Overview of the functionality
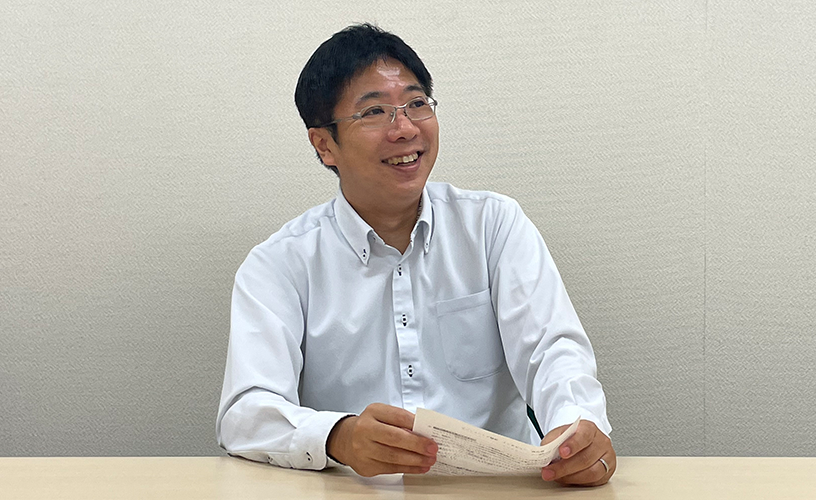
Interview with Mr. Junya Morita
This function enables positioning to be analyzed by extracting reference points for the positioning from previous mammography images that are compared with predetermined values.
Positioning is objectively evaluated at each imaging session, thereby supporting technologists in improving their skills to capture images that facilitate diagnosis.
With this function, the reference points of the pectoralis major muscle and nipple position for positioning are extracted from past images using AI technology*1, and index values for positioning such as distance from the reference point to the nipple/pectoralis major muscle is calculated.
By comparing these index values with the predetermined values, positioning can be quantitatively
evaluated.
- *1 The extraction of the reference point of the pectoralis major muscle and nipple position for positioning was designed using an AI technology called “deep learning.” There is no automatic change in performance or accuracy of the system after installation.
Impetus for the development of the positioning analysis
I have been involved in the development of image processing for mammography for approximately 17 years. During this time, I had the opportunity to work with doctors on image evaluation, and learned that the quality of positioning can affect the quality of images, which makes a significant impact on the detection of lesions.
Image design and processing are also important factors to improve the quality of diagnosis.
However, an image obtained with poor positioning quality cannot facilitate the detection of lesions, no matter how hard we try to enhance the image. Hence, we considered that we could better contribute to the provision of images that facilitate diagnosis by supporting improvements in positioning techniques.
Meanwhile, when evaluating images, images from around the country as well as around the world are used.
We have learned that breast size and mammary gland volume vary greatly among individuals and races, and that positioning criteria vary widely from country to country and facility to facility. This led us to recognize the need for an objective index to evaluate the quality of positioning, and we began to search for a solution.
Evaluating captured images based on numerical data - Identifying technologist’s
weak points and habits
By establishing indices for evaluating positioning, technologists’ weak points and habits can be identified to help them improve their positioning techniques.
Through quantitative evaluation, they will be able to clearly understand aspects they would not notice in subjective evaluation of individual images.
Numerical data can be accumulated statistically, and the results of analysis utilized to further improve positioning techniques.
Path to product launch
To quantify positioning items from mammography images, I thought it was necessary to ensure highly accurate recognition of the breast structure for image analysis.
In particular, a major challenge in development was setting up the parameters used to identify nipples, pectoralis major muscle, etc. for the evaluation of positioning.
Although an AI technique called “deep learning” was introduced to ascertain the structure of the breast, it was not easy to accommodate various breast shapes and differences in the way the images were being taken, due to differences in facilities and patients.
For example, the nipple is an area that frequently cannot be identified on an image. Sometimes the nipple was not captured in the image even if it was well positioned, and sometimes it was difficult to recognize the nipple because it overlapped with the breast.
Also, when identifying the pectoralis major muscle, structures similar to the pectoralis major muscle were captured, and, in many cases, it was not clear which one should be correctly identified as the pectoralis major muscle.
In images obtained overseas in particular, the latissimus dorsi muscle and twisting of skin were often captured. Therefore, we started by consulting with doctors and technologists to find out how to recognize the pectoralis major muscle.
Determining appropriate factors for evaluating positioning was another major challenge.
Doctors and technologists have expertise to instantly evaluate the quality of positioning by looking at images.
To achieve this technically, we had to work on breaking it down into a language. We looked at various images and decided on evaluation items based on expert opinions.
In addition, in anticipation of worldwide deployment, we tried to include evaluation items according to the guidelines of individual countries.
For example, the measurement of the posterior nipple line (PNL), “the PNL (distance from chest wall edge to nipple) on the mediolateral oblique (MLO) view should be no more than 1 cm longer than the PNL on the craniocaudal (CC) view of the same breast” is not specifically defined in the Japanese guidelines.
As such, we have included a function to turn on/off each item.
Through these efforts, we have succeeded in commercializing a highly useful positioning analysis tool, although the path to commercialization was fraught with challenges.
Utilization of function
- Added technical feedback function in addition to positioning evaluation items
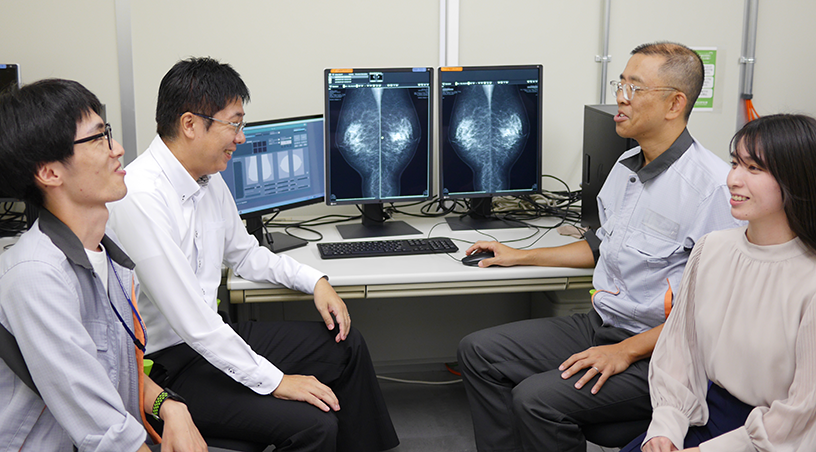
In addition to the evaluation items, the positioning analysis system has an additional function to record and share information about patient’s pain and ease of imaging.
This makes it easy to pass on the previous imaging situation to the next imaging session.
Sharing such information on imaging situations can improve not only the accuracy of positioning, but also the quality of communication with patients.
To cite an example, if we know that a patient strongly complained about pain during the previous session, we can care for the patient’s pain before imaging, thereby making the patient feel more comfortable with the imaging.
We expect this system will improve the quality of mammography and bring about benefits to both patients and technologists.
It is hoped that the functions of the system will be fully utilized to improve techniques and to enhance the attitude of care for patients, which will lead to better diagnosis.
In closing
The positioning analysis system enables objective evaluation of positioning of captured images based on numerical data. Technologists’ weak points and habits can be identified to help them improve their positioning techniques.
In addition, along with positioning analysis, patient information can be recorded and shared, which we believe will enhance the quality of the mammography.
We sincerely hope this system will benefit both healthcare professionals and patients, and contribute to improving the quality of mammography.